Published:
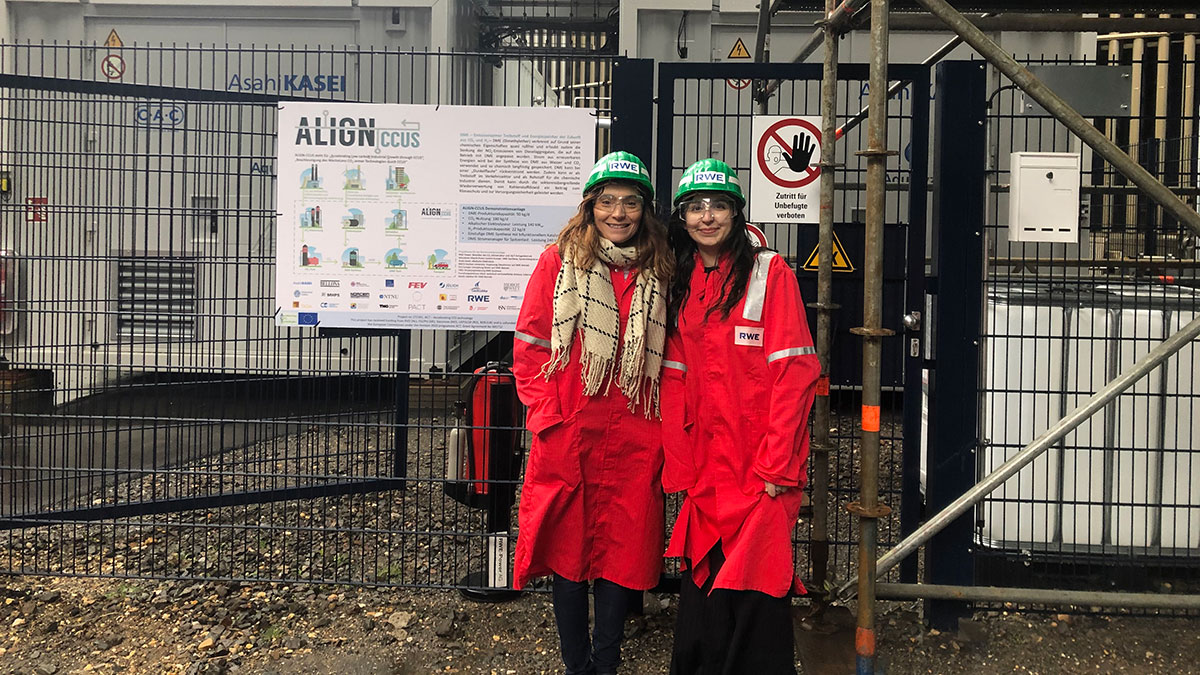
Scientists have developed a machine learning approach to accurately predict potentially harmful amine emissions from carbon capture plants.
The team from Heriot-Watt University and the Swiss Federal Institute of Technology Lausanne (EPFL) have had their findings published in the academic journal Science Advances.
Global warming is partly due to the vast amount of carbon dioxide released mostly through power generation and industrial processes, such as producing steel and cement. For a while now, chemical engineers have been exploring carbon capture, a process that can separate carbon dioxide and store it in ways that keep it out of the atmosphere.
This is carried out in dedicated carbon capture plants, which involves amines as part of its chemical process. These compounds are already used to capture carbon dioxide from natural gas processing and refining plants as well as in certain pharmaceuticals, epoxy resins, and dyes.
The problem is that amines could also be potentially harmful to the environment as well as a health hazard, making it essential to mitigate their impact. This requires accurate monitoring and predicting of a plant's amine emissions, which has proven to be no easy feat since carbon capture plants are complex and differ from one another.
A group of scientists has come up with a machine learning solution for forecasting amine emissions from carbon capture plants using experimental data from a stress test at a plant in Germany. The work was led by the groups of Professor Susana Garcia at The Research Centre for Carbon Solutions (RCCS) at Heriot-Watt University in Scotland and Professor Berend Smit at EPFL's School of Basic Sciences in Switzerland.
The experiments were done in Niederhauẞen, on one of the largest coal-fired power plants in Germany. And from this power plant, a slipstream is sent into a carbon capture pilot plant, where the next generation of amine solution has been tested for over a year.
Professor Susana Garcia, Associate Director of Carbon Capture and Storage at RCCS, together with the plant's owner, RWE, and TNO in the Netherlands, developed a stress test to study amine emissions under different process conditions. Professor Garcia describes how the test went: “We developed an experimental campaign to understand how and when amine emissions would be generated. But some of our experiments also caused interventions of the plant's operators to ensure the plant was operating safely.”
These interventions led to the question of how to interpret the data. Are the amine emissions the result of the stress test itself, or have the interventions of the operators indirectly affected the emissions? This was further complicated by the general lack of understanding of the mechanisms behind amine emissions. To solve that challenge, the team of researchers developed a machine learning approach that turned the amine emissions puzzle into a pattern-recognition problem. In that way, they could predict the emissions caused by the interventions of the operators and then disentangle them from those induced by the stress test. In addition, they could use the model to run all kinds of scenarios on reducing these emissions.
The conclusion was described as “surprising”. As it turned out, the pilot plant had been designed for pure amine, but the measuring experiments were carried out on a mixture of two amines: 2-amino-2-methyl-1-propanol and piperazine (CESAR1). The scientists found out that those two amines actually respond in opposite ways: reducing the emission of one actually increases the emissions of the other.
Professor Garcia concludes: “The potential impact of this work is enormous. We have been able to successfully forecast amine emissions from carbon capture plants that use the most advanced and latest state-of-the art amine-based technologies. This type of forecasting proved to be very challenging with any of the conventional approaches, so it may change the way we operate industrial plants. This work also highlights the key importance of having multidisciplinary teams if we want to successfully address global challenges."